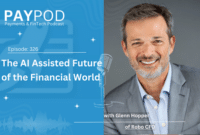
The AI-Assisted Future of the Financial World with Glenn Hopper of Robo CFO
Episode Overview
Episode Topic:
Welcome to an insightful episode of PayPod. We engage Glenn Hopper, the creator of Robo CFO, in the discussion the focus is on the intersection of finance, AI, and innovation. He shares insights into the evolution of his career and the inception of Robo CFO. The conversation delves into the vast potential for AI in the financial world and why it stands as prime ground for technological innovation. The episode also explores the workings of GPTs (Generative Pre-trained Transformers) and their role in reshaping the financial landscape.
Lessons You’ll Learn:
There is a profound understanding of how GPTs function and the diverse applications they have in the financial sector. Glenn Hopper shares the intricacies of creating specialized AI professionals tailored for distinct financial tasks. The discussion unfolds the benefits of using GPTs and provides insights into the creation process, feedback loops, and continuous evolution of these AI tools. Moreover, the episode addresses concerns related to job loss in the face of AI advancements, offering nuanced perspectives on the ongoing debate.
About Our Guest:
Glenn Hopper, the mastermind behind Robo CFO, takes center stage as the guest in this episode. With a rich background in finance and a keen eye for technological advancements, Glenn shares his journey and the pivotal moments that led to the development of Robo CFO. Glenn’s expertise and his thoughts on the evolving role of AI in reshaping the financial landscape is an exclusive lore.
Topics Covered:
The episode covers a wide array of topics, from the genesis of Robo CFO to the implications of AI in the financial industry. Listeners will gain insights into the distinctive features of GPTs and their real-world applications. Glenn delves into the potential beneficiaries of these AI tools, the creation process behind each specialized professional, and the dynamic nature of feedback and updates. The episode also navigates the challenges and opportunities in implementing AI technologies within businesses, providing valuable guidance for CEOs and companies aiming to integrate these transformative tools.
Our Guest: Glenn Hopper- Thought Leader in Fintech with AI Job Impact Insigts
Glenn Hopper, the visionary creator of Robo CFO, brings a wealth of financial expertise and technological acumen to the forefront of this podcast episode. With a distinguished career marked by a fusion of financial acuity and technological innovation, Glenn has become a trailblazer in the field of AI-driven financial solutions. His journey unfolds from the early days of his career, navigating the realms of financial accounting, principles of finance, and business analytics. Glenn’s pivotal realization that the financial domain could benefit immensely from AI and advanced technologies laid the foundation for the inception of Robo CFO.
As the founder of Robo CFO, Glenn has embarked on a mission to redefine the role of AI in finance, specifically targeting the areas of financial forecasting, time series analysis, and business analytics. His unique approach involves leveraging Generative Pre-trained Transformers (GPTs) to create specialized AI professionals tailored for distinct financial tasks. Glenn’s commitment to demystifying the complexities of AI is evident in his engagement with the audience, sharing insights into the workings of GPTs and their potential applications in the financial sector. Notably, he emphasizes the importance of reskilling and upskilling teams in preparation for the transformative wave of AI, positioning Robo CFO as a pioneer in the evolving landscape of financial technology.
In addition to his groundbreaking work with Robo CFO, Glenn is an active contributor to the discourse on AI and its implications for job roles in the financial industry. Addressing concerns about job loss due to AI advancements, Glenn provides nuanced perspectives on how technology can coexist with human expertise. His vision extends beyond the present, urging businesses to focus on data maturity and laying the groundwork for seamless integration of AI tools. Through his role as a thought leader and entrepreneur, Glenn Hopper continues to shape the narrative around AI in finance, guiding companies toward a future where humans and AI collaboratively drive financial innovation.
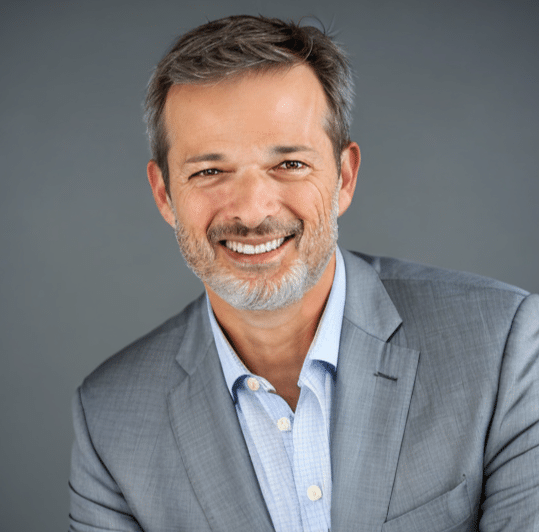
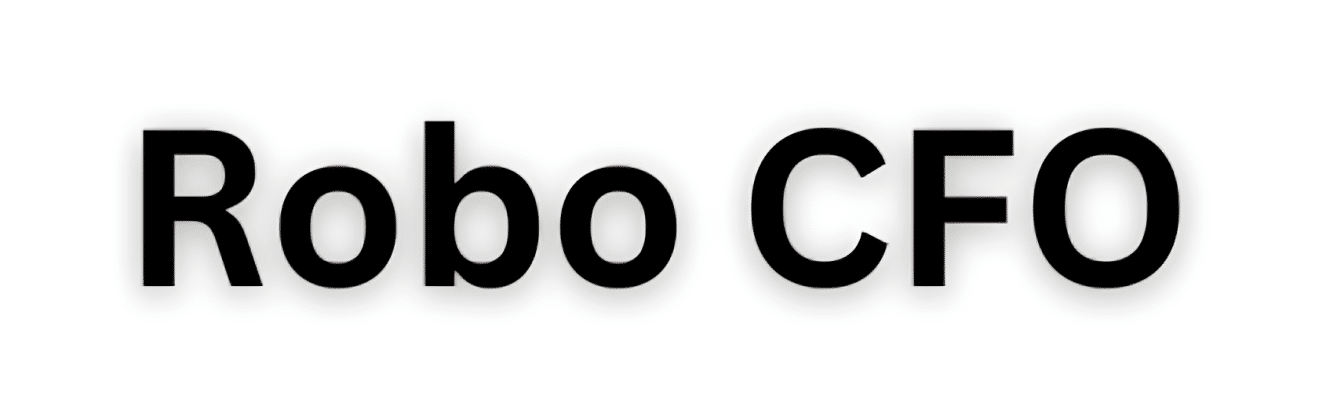
Episode Transcript
Glenn Hopper: I think of them as highly intelligent but very green. Interns. Like they just come straight out of the best business or accounting program in the world. They know everything. They’ve got a perfect memory, but they just don’t have the experience in the world so they can still give you wrong answers. So that’s not a knock against my products in particular. It’s a problem across the realm with generative AI, but it is something to keep in mind. Don’t lean too heavily on these tools to do your day job, but they’re great assistants.
Jacob Hollabaugh: Welcome to PayPod, the payments industry podcast. Each week, we’ll bring you in-depth conversations with leaders who are shaping the payments and fintech world, from payment processing to risk management, and from new technology to entirely new payment types. If you want to know what’s happening in the world of fintech and payments, you’re in the right place. Hello, everyone. Welcome to PayPod. I’m your host, Jacob Hollabaugh. And today on the show, we are going to be talking about how new technologies will shape the future of work in the financial world, with a focus on AI and in particular AI bots such as GPT. Long-time listeners of the show know my personal affinity for the AI space. Given the same tired AI-related jokes and Segways that I tend to use most episodes. But today I don’t have to use any of them because the whole episode is going to pretty much be about that topic. So I’m very excited to get to spend this whole episode looking into this world. Join me for this conversation. I am very pleased to have Glenn Hopper, founder, and CEO of Robo CFO, a company which consists of a collection of specialized AI professionals that help financial professionals revolutionize how they handle finance and accounting, blending advanced technology with expert knowledge. Glenn, welcome to the show. Thank you so much for joining me today.
Glenn Hopper: Hey, Jacob. Thanks for having me here. yeah, we’re talking about my favorite topic. I hope you do keep dropping eye jokes. And I’ll try to throw in some as well. So we.
Jacob Hollabaugh: Well, they may be mostly up to you. Mine aren’t great jokes. I pretty much just had 2 or 3 segways that I used for the last calendar year, plus of ways to get every guest to ask about the joke, I guess that 2023 was the year of AI. So I had to ask every guest, no matter what they were doing, what we were talking about. I was like, we have to at least mix in a little I talk here, but today we’re going to mix in all of it because that is the main focus of what you’ve got going on. So give me and the listeners some background here before we actually dive into the different parts of Robo CFO and what GPTs are and the whole thing. Give me some background. What’s the kind of high-level path of your career looked like, and how did you end up in a spot in a position where the idea for building out Robo CFO came to fruition?
Glenn Hopper: Sure. So there’s a quote that, I don’t know if it’s exactly my origin story, but it explains my motivation for getting interested in tech very early in my finance career. The quote, I think it’s often misattributed to either Steve Jobs or Bill Gates. Somebody probably says, Mark Twain said it, I don’t, but the quote is actually, I think it was someone from the auto industry in like the 40s or 50s talking to Congress. And, they were talking about production and efficiency, and uh what this automotive leader said was, if you want to find the most efficient way to do a job, give it to a lazy person. so from early on in my finance and accounting career, I saw a lot of work that was just really repetitive and really manual. And where in my whole career it’s been, how can I make my outputs greater than my inputs? And if I’m wasting a lot of time on data entry and just sort of the mundane parts of the finance and accounting world, then I’m not going to be able to really add any value anywhere. So, throughout my career and that involved coming up through FP&A and being a startup CFO for 15 years and doing the consulting work I do now, I know that no one’s going to hire me to just, I’m just some kind of hourly output. So from the very beginning, I’ve been using technology to automate things, and it has just been in my own best interest to find ways to streamline processes. And interestingly, when you automate and streamline processes, one of the byproducts of that is you have better data. So as AI and machine learning started getting more and more commonplace and more broadly available, I just started finding more and more ways to streamline workflow. So that kind of just now you get into a world of generative AI, and it’s kind of my time right now. It’s like, this is what I’ve been saying.
Jacob Hollabaugh: Yeah, it makes sense. And it’s now the time for folks that were maybe interested in it but didn’t have the tech technical knowledge to actually go build something in the past where now the main advancement really of a lot of these tools is just the accessibility to use them as much as what they actually do. And kind of what you said it there at the end reminds me of all the conversations I’ve had on this podcast, maybe the main takeaway if I was to like, kind of jot down what are the most highest level truths about this world of financial technology and payments and everything that we talk about on the show, one of the main ones has been how ripe the financial world has been for technological innovation, and certainly in the last couple of years, as a reference, I’ve made all the AI jokes and asked everyone about AI because it seemed like the financial industry was maybe the most ripe of any industry for AI in particular, to be used to build efficiencies into systems and structures. Do you agree with that statement, kind of that this industry, all industries can use all these technologies, of course, but that maybe the financial world being so data heavy and everything made it kind of the optimal proving ground, breeding ground, testing ground, if you will, for new technology. And what is it about the industry that kind of gives it that is it just simply that it’s based on a bunch of data and this stuff all is run off of data and that’s the whole story or is there a little more to it?
Glenn Hopper: Yeah. So great question. And, as you were first posing it, I was thinking just straight down one line, but now I’ve got a bifurcated response here. And let me get if you know, with your audience being financed and accounting people, we are risk averse by nature. And I think this we should be that’s part of our job. We’re not going to be gambling the company payroll at the relabel or whatever. So yes, there is reason to understand something and technology space, understand what it is, how it works, what the drawbacks are and all that. So I don’t think I’m ever going to advocate for finance and accounting to be on the bleeding edge of technology. We don’t want to be with all the problems with hallucinations, with these large language models in the last year, we don’t want to be signing off on financial statements that first Adopters.
Jacob Hollabaugh: Maybe right behind the first adopters. We cannot be the ones proving, finding the bugs, so to speak.
Glenn Hopper: Exactly. So I will now semicolon. However, we do have so much data. And really, the evolution of the CFO’s office has been significant. So my first CFO gig was in 2007, I guess. And thinking about even from 2007 to today, with the increase in the amount of data out there, the greater the expectation for like real-time close of or at least very fast close every month for the financial statements and for all the work that we do with all this data out there and the data being accessible by other groups, us having to define where or to add value, rather just reporting something that happened in the past that’s not really adding a lot of value anymore. So the shift in the finance function has gone more and more forward-looking. You know, forecasts are building out ROIs for projects and being able to justify things and being able to explain variances. So we have been gathering data for 20+ years now in finance and accounting. But somehow and this is what I was thinking when you were asking the question, somehow sales and marketing have passed us and they’re like the leading users of machine learning and AI. They’re a b testing for their, I mean, somehow sales and marketing became the data experts. And when I see that just time and time again in companies, I feel like. But finance and accounting people were the original like bi people. We’re analysts at our core, how did we get passed by all this? And I think it’s that risk-averse nature. And I think with sales and marketing, it’s they’re more out there on the leading edge. They’re trying to come up with the latest product and everything. And I think when you’re arguing for a budget, something that grows the top line, it is going to get more attention than finance and accounting that’s seen in the back office and as a cost center a lot of times. So, yeah. So we are ripe for it. We have a lot of data. The role is changing every day. This is just going to change it more. yeah. That’s I mean that’s where we are now. And it’s going to be very interesting to see as these tools become more widely available, not just to the giant enterprise businesses, but to the SMB space, which is where I’ve spent my career.
Jacob Hollabaugh: Yeah. And all the way down to the individual level. So before we actually get to the details of Robo CFO, I do feel like it’s necessary to at least ask you to give listeners a bit of an explainer on GPTs. I think everyone at this point, I would assume anyone listening has at minimum heard of ChatGPT if not tried it out, or similar AI bots out in recent times and hopefully many listening are leveraging them to great benefit, using them for all the many things and efficiencies and benefits they can bring to our life. But the idea of GPTs are a little bit more recent. I forget, exactly, the announcement was only what last fall? And they rolled out fairly recently. Still here speaking you know to you today in the end of January here 2024. but they are a little more new. So could you give a little overview as to what it means when we refer to GPTs when they did come about, who can make them, and how the kind of marketplace for them worked in these early days of them?
Glenn Hopper: Sure, sure. And to first answer your question, I’m not sure what a GPT is. And I’ll say, here’s what I mean by that. So GPT, what it is, OpenAI is terrible at naming products. So GPT stands for generative pre-trained transformer, which is what the model is that the LM is built on. So the fact now that anyone can build a GPT. Well, we’re not building our own generative pre-trained transformers. So I don’t know why they call them, what they do. But in the context of what we’re talking about today, a GPT is, customized. And we can we can get into detail on this. But the easiest way to say what a GPT is, is it’s something that’s built on top of a large language model as the foundation model, as the platform that lets anyone and we can talk specifically about when I say anyone, I mean, if you can speak English or whatever your natural language is, you can build a GPT because it’s that’s one of the amazing benefits of generative AI is it kind of turns natural language into essentially another programming language because it does all the work for you behind the scenes. But what GPTs allow you to do is take their foundation model and then fine-tune it with either prompt engineering and or adding your own custom documents. So this is where they get really interesting, your own documents. In my case, I was training these bots on finance and accounting practices, everything from audit to, ESG to FP&A. So I built custom bots that had very specific instructions, but I also sent them back to school by uploading a bunch of open-source textbooks that would train them on accounting principles, on time series analysis, on EOB compliance. But the great thing is, when I built my first one was before GPTs were rolled out, it was just I built an app in Python that I had ChatGPT write a lot of the code for me too, which was amazing. But It took a long time, I’m going to say probably 15 to 20 hours of development time to build this thing. But I trained an auditor on, everything from SEC regulations, PCAOB, basically anything that an auditor would have to be trained on. I uploaded it, and let the model refer to that. but that took forever and it was clunky and it was not nearly as useful as GPT is. The same week that I rolled that out, like three days later was OpenAI’s Dev Day, where they rolled out the GPT. But I wasn’t even mad because I thought I built this prototype and it kind of works. It doesn’t work like I want it to. It’s clunky, it’s not production-ready. And then that weekend after Dev Day, as soon as they were available, I built out half a dozen of them, and I’ve just been adding to them ever since.
Jacob Hollabaugh: Yeah, I love that. And I like to use the analogy for people. It’s kind of how it was first explained to me, and I think they even used it during that dev day when kind of doing the explanation of the ones you’re going to be talking about are all built within ChatGPT itself. So you have to log into ChatGPT and it’s kind of like the App Store almost for ChatGPT that you can go in, search for things, find them and install them and what they are is then what exactly what you said that you’ve gone and taken everything ChatGPT itself is built on, but added your own inputs to it, your own parameters, your own prompts, and then put that out on the kind of app marketplace, if you will, to say, “I’ve built this, instead of you just going to base ChatGPT to ask your auditing questions, I’ve built the best version of it. I’ve already done all the prompts, added the inputs, everything you would need for you now to go to this specific GPT that you would download and you install within ChatGPT.” So it’s kind of like the App Store a little bit within them, although I’m sure many of their competitors will now start doing similar things. And as you said I love the experience that you had got to kind of build one solo out on the side, right up and then leading to, I’m sure that at least made the legwork of what am I going to now put into this now that I can build it within ChatGPT a little bit easier, hopefully for you. So give me the rundown then. Right now, who are with Robo CFO? You’ve got a suite of different AI professionals, Kind of give me the high level who are some of these different GPTs that you’ve built, these different AI professionals. And what are each of them kind of built for?
Glenn Hopper: Sure, sure. So let me start with our fearless leader. Rosie, the Robo CFO. Now Rosie is trained with all these depending on what I wanted them to do. It’s all about picking what data you train them on, what you give them to refer to. So, as the CFO, Rosie had to have a broad range of knowledge. So everything from accounting to treasury to public markets to private equity. I mean, she’s got a bunch of text that, I’m anthropomorphizing here because I’ve given them people names. So I’m going to talk about it.
Jacob Hollabaugh: Yeah, I do encourage anyone listening or watching to the, the link to the site will be in the show notes, and you definitely got to go check out who these folks are and their images that you’ve done a masterful job, and I’m guessing they do look if I were to guess, maybe another AI bot used potentially to create the images in these AI professionals for you. But they’re fantastic artwork, fantastic people. So I encourage people to go look at who we’re talking about as you’re explaining them here.
Glenn Hopper: Yeah. Thank you. And yes, it was I’m trying to do everything with AI these days. You know, it’s like, it’s that lazy person thing. Where can I save time? And I certainly couldn’t have. It would have been very expensive to pay for the design of all these images and everything. It just with generative AI, you just crank them out. So a lot of efficiencies this year,
Jacob Hollabaugh: I’m sure, there’s like at least one teacher in your past that you could go back to and say, you told me being lazy would never be an asset, but I found a way. I’ve built an entire career off of this. What little did you know?
Glenn Hopper: Yeah, exactly. So, I want to come back to Rosie, though, because I have longer firm plans for her. I. There’s a lot of work in the space around agents, and I don’t know how deeply you’ve gotten into it, and my ultimate goal with Rosie is that she is more self-taught and is able to interact with the other bots, the functionality I’ve been there that there’s more than 100 different agent type things out on hugging face, and on other websites where this is tracked, they’re just not quite ready for prime time. But that’s my ultimate goal with her. So, and I won’t go into that level of detail of everyone else, but just to give you an idea of the types of bots that I’ve created. So I’ve got if I’ve got the CFO, I’ve got to have a controller. So we have one of these GPTs that’s trying to be a controller. I have an auditor. I have I think I mentioned before, an ESG researcher who specializes just in ESG. I’ve got an SEC director who understands all the ins and outs of Qs and Ks and S-1s and F1s and public filings and everything you need on the public side. I have a favorite one. And if we want to talk more about the training, she’s the one I’d love to talk about because she’s got a really because this is my true love and finance is doing for financial planning and analysis. So I spent more time on her than I did on some of the others. But, that’s Francine, the FP&A guru. And then I’ve got a treasurer, an economist, and an IFRS specialist. One of the bots is focused on just M&A. I’ve got a stock analyst and then I’ve got because I write a lot of articles on this. I built a GPT that is thinking of him as like an investor relations bot. He’s trained in writing journalistic style. And so I use him if I have to write an article. He’s like, not my editor. You got to have your own voice when you write. I would never tell anybody. Just turn over all your writing to AI because it’s got its own cliches and everything it uses. But it’s great at outlining, coming up with ideas and all that. So it’s like a collaborative partner that I use. And there are a couple others that I use daily just that I haven’t made public because I just use them as worksheets for me.
Jacob Hollabaugh: Yeah. So I do want I’ll come back to Francine, there to use as an example. I do want to ask a little about the creation process and inputs and stuff, but before I do that, I think it’ll be helpful for people to know. Is there anyone in particular that you have in mind when building these? As far as you know, obviously, the goal is broadly anyone who does works in those roles. If you’re a controller at any company, any industry, any type of way, you know you could benefit from it. Of course. So broadly, kind of everyone. But I’m wondering, was there any more specific set of folks who maybe you mentioned small SMBs earlier on anyone, whether it’s a specific role, leadership role within a company, whether it’s a specific industry-specific company size, where the work you’re doing might benefit from these the most. Are there any specific folks you had in mind for these, or was it just broadly anyone doing this work, no matter the industry, no matter the size, the scope, etc.?
Glenn Hopper: So let me caveat this first. And I think it’s always important for me to say this. these GPTs are great. I’m in the trust but verify phase with them, because there are still this pre-training that I’ve done in this fine-tuning, and by making them default first to the data sources that I give them versus the, broader source that it was trained on helps a little bit. But We’re still definitely in trust but verify on them. So that caveat aside, the second caveat I want to put out here is. So unless you’re in and we’re sticking to GPT here because that’s where OpenAI is where these are. So apply this to barred or anthropic or any of the other models out there as well. But I’m cautioning everyone not to put PII, personally identifiable information, or proprietary information into the web-based chat tools out there. you know, it’s just like if you put that information anywhere, anywhere else in the cloud you have to be cautious with it. And whatever you’re using, if it’s financial data, you want to be sure it’s SoC compliant. and all that. but also in those publicly available platforms, the warning that comes up is your data may be used for training data. Now, we could get into the likelihood when something is trained. I mean, the amount of data that these models are trained on, it’s the only way to think of it that would make even close to the amount of sense of understanding how much data went into this.
Glenn Hopper: Think of them. They’ve basically read the entire internet. I mean, that’s the really the good bad everything out there. So the chance that your data would filter through all that in a, in future training iteration, it’s not very high, but it’s non-zero. So what we’re going to see a lot of in the next I think and it’s happening quickly and already. So first there was OpenAI for enterprise, which I think the entry-level cost for that was 125 to 150 grand for a business just to use that out of the gates. But if you had open AI for enterprise, your data wouldn’t be used for training data, it would be stored. It would be in its own walled-off section. That’s great. but too expensive for most SMBs in the in last couple of weeks, they rolled out, this team account, which is only $30 per user, the same kind of thing. Your data is not going to be used for training, so every company is going to have to come up with their own AI policy and what they’re using and what they’re not. But here’s the danger, and this is where I’m getting to who will who will use these if companies don’t have a policy or even if they do if it’s too locked down, you’re going to find the lazy people appointed myself, who find these shortcuts, that they can use these tools and work much faster, and they’re out there very recently that show how much more productive you can be with it. So, Risk averse is fine. We can’t bury our heads in the sand on this. It’s moving too quickly. People are using it. So all that said, I feel like that should be the fast-talking legal disclaimer part before I say it. But as I alluded to earlier, I’ve been one of these citizen developers from way back, and if I couldn’t get the support that I needed from it, I was building stuff on my own. And that sort of drove the development of tools like this. but if you’re doing client work or work in your company, you want to be sure you’re operating within the confines of the company. But I think about how much time I work with a lot of technical accountants, and we get way down in the weeds questions and the amount of time it takes to research those and come back with an answer that’s very inefficient. but if you had a tool that was. Designed to understand your question contextually, was fine-tuned on the same data that you’d be going to research for your answer, and could quickly give you an answer back with citations of where it came from. I mean, think about the amount of time saved there. Or if you’re you’re an FP&A person and you’re trying to do a forecast to modify the forecast for the next six months, and you’ve got some different scenarios you want to run and you can do it in Excel. And there’s other software you can do it in, but it you know, it takes a lot of time, even with a template you know, it still takes time to do it. With generative AI, you could plug in all of your information, and have it do a Monte Carlo simulation with ten run the model 10,000 times and much quicker than if you were trying to do it yourself. So there’s there are ways that sort of the individuals within a company can use it, of course, within the confines of the company. I don’t want any of your listeners to get in trouble for going out, going rogue, and using something like this, but it’s, and really, right now, I mean, I’m not. I do not see any of these GPTs taking anyone’s job. These are our tools to help you. But technology is moving so fast. I mean, and that’s what you could do a whole episode on the labor replacement from AI. But, right now they’re just tools to help. Don’t lean on them too heavily. I think of them as highly intelligent, but very green interns. Like, they just come straight out of the best business or accounting program in the world. They know everything. They’ve got a perfect memory, but it they they just don’t have the experience in the world. So they can still give you wrong answers. So that’s not a knock against my products in particular. I mean, it’s a problem across the realm with generative AI, but it is something to keep in mind. Don’t lean too heavily on these tools to do your day job. But they’re great assistants.
Jacob Hollabaugh: Yeah, absolutely. So take me then to what was the kind of creation process like. And you can use Francine as kind of the example there of what were some of the like types of inputs in where you did you have a bunch of your own data sets? Was it more prompt-based? What was kind of the mix of inputs? You went into the creation process for each one of these?
Glenn Hopper: Sure. And so when I built the tool before GPT wearable, I went and found some open a bunch of text sources. So just went online and searched for open, open source textbooks and got what I could because there’s there’s the whole copyright thing. And if you’re using something that isn’t available so you can use your own data. But there whatever data you use to train these things right now, I think if someone could go and ask, what’s your training data? And it will tell them, and I think in some cases even let them download the data. So again, you have to be careful with all this. We’re kind of in the we’re on the bleeding edge right now. But when I built Francine I thought about like I said, FP&A is my favorite part of finance. I love building complex models and you know, doing forecasts that are based on more than just doing a linear regression and carrying out what your current run rate is. so I started thinking about what are some of the tools that I use. And. The interesting thing before you make a GPT, that if the base model has read the entire internet, then they’ve been exposed to this data before. But it doesn’t. They’re not domain experts and they don’t they’re not going to default to automatically looking in the finance and accounting realm. So the first thing I did was I wanted to prime all these bots to be thinking with finance and accounting. So the first and I’m looking now at what I put in there. So the first, text I put in was a textbook on financial analysis, one on statistics.
Glenn Hopper: I put a copy of my book in there because I don’t know, but yeah, yeah. financial accounting, principles of finance and then a business analytics book. So I thought, here’s sort of your domain expertise. Look here first before you go out to the broader your broader knowledge base, and you’ve got, your finance in accounting base here. and then I thought if I was going to do advanced modeling, what would I need to add to it? So more business analytics texts, a machine learning textbook, a time series, an analysis textbook, and a bunch of white papers. So it’s it’s keeping it. I mean, by uploading all these documents, it’s using retrieval, augmented generation, where instead of just going straight to the base model, it’s looking in these first. But it’s by even just having this text in there, it has the model already thinking in sort of the finance and accounting and forecasting and time series analysis. And then the rest of it was custom instructions that are just in plain English. I say you know, here’s what you’re good at. Here’s how I want you to interact with people. And it’s probably 3 or 4 paragraphs of tuning. But unlike the first project I did that I had to do everything in Python. When you’re building these GPTs right now, they’ve got an interface. You just type text in and it helps refine for you, and it’ll even name your GPT. It’ll create an image for your GPT. I ended up using my own, not the default ones, but it’s they’re making this as easy as possible for anyone, regardless of your coding background, to be able to build these tools.
Jacob Hollabaugh: Yeah, it is really amazing. And we’ve kind of referenced that accessibility part early on in our conversation that is the technology continues to get amazing. the outputs that they can create and everything. But the biggest unlock in reality over the last few years is really the accessibility to use those things. Yes, the outputs have gotten more impressive and better and are built on bigger data sets and everything else but the accessibility piece. It really just kind of opened the door to a big part of this already existed, just only a few people got to use it or interact with it or knew how to or had the technical skills to do so. And now all of us can. so anyone listening, if you’ve I before we got on this call, I’d reference to you. Glenn. You know, I have a few ideas in my own head of GPT. I could build as someone who’s a podcast host. There isn’t. I’ve been checking out there some people who have made ones, but like, things like helping me do my research on my guests and the companies and things I’m talking to that can really. Yes, I could just go type that into ChatGpt based on its own, the results having done that before, are not that great. But if I took the time to kind of build-up, here are the inputs you should be kind of using as your base, I think something like that could be built. So anyone listening, whatever you have whatever the little parts of your jobs think like the lazy person would, as we’ve said a few times of like, where could these things help me? And maybe you’ve tried one before and were like, I didn’t really love the results. Well, maybe there’s a more updated version. Maybe you could create that version, because that accessibility being pretty much eliminated or opened up to everyone is, is pretty amazing. One thing you referenced a few minutes ago is that these are certainly not meant to replace anyone’s work, not meant to replace your own work in any way, but they’re meant to be an aid. I really loved your kind of the greenest intern but the most brilliant intern ever. But no experience. It was a really good analogy, but you did reference the kind of job loss fear that is out there. And so we don’t we won’t do the whole episode here on it. But I do want to ask just kind of about that topic in general that I think that is probably the number one fear. I guess maybe the biggest fear is taking over the world or something. So maybe right below that Armageddon. Yeah. The biggest the first fear for most people is am I ever going to lose my job? Or if I use something like this, am I going to end up? Maybe if I start incorporating a bunch of this stuff, I work with a team of five people and I’m the leader of the team.
Jacob Hollabaugh: Am I not going to lose my job? But if I end up going to be like, well, I don’t need three of the five of you anymore because we’re all so much more efficient or better at this. So have you had maybe any pushback having built these, in, in, in any way, or just? In general, kind of. How do you think about the idea of yes, these right now, what you’ve built are meant to just be an assistant? And I’ve always been a big believer in we have to learn to work with them. We won’t be a little less fearful of them replacing us, but it’s just kind of a new skill set. There’s not really a question in all of that, I guess, but just generally kind of if you were to give your one answer. Thoughts on this versus the full podcast episode, thoughts on it? What are your thoughts around kind of the job loss aspect of it? And the more they help us be efficient, the less we all are needed or the less of us that are potentially needed to run these companies and do these jobs.
Glenn Hopper: So my first thought on it is every technological revolution or industrial revolution, every big change has led to a change in the way people work and the kind of jobs they have and where they work. but the economy, the economy moves on. New jobs are out there. I mean, so we’re in 2024 now. think back to, I don’t know early 20th century, 120 years ago, something like 75, 80% of the population in the US, worked in agriculture. And then as as technology changed and things became automated there. And then, I mean, you can think about the manufacturing sector and all the jobs that happen there. Well, nobody knew what an online content manager was back then or whatever all the jobs that have come out and since then. So there’s been a shift. Now, the better our technology gets, maybe first it came for you know, the mechanical looms, and then it was you know farm work and, and all the technology that has come before. So, yes, there are a lot of jobs that have changed, but new jobs have come in their place. But the better our technology gets, the more potential jobs could be replaced. And I think all of us white-collar employees thought, well, we’re going to be the last ones. They’re going to have robots doing stuff before this. And now with AI and with generative AI in particular, it’s like, well, this seems like it’s doing a pretty good job of at least proximate to, the work that I do. So, I mean, the work world is going to change. This feels like I’m old. So I remember in the early days of the internet from the AOL and Prodigy dial-up and all that, and it just seemed gimmicky at the time. No idea where it was going to go, but you knew something big. And we’re at another one of those sort of precipice moments right now. So jobs are going to change in finance and accounting. Right now we have a shortage, CPAs are retiring and that funnel is not being filled. So, people who may be used to going into accounting, have the same kind of skill set and the same sort of way of thinking. Instead of going into accounting. Now they’re taking these data science jobs and business analysts and some going even into finance rather than just strict accounting. So what we may see in a short time is we’re going to get we’re going to accelerate our adoption of AI because we can’t find these people to come in and do the sort of base-level jobs. So I’ll just close with one more thought on that, and it’s one of my favorite quotes. I use it all the time. It’s a quote from a guy named Cliff Stoll. but it is. Data is not information. Information is not knowledge, Knowledge is not wisdom, and Wisdom is not understanding. And the reason I use that quote all the time is because I see AI technology moving up that ladder. So we have to stay ahead of where AI is and that ladder and figure out where we’re providing value. So our jobs are going to change. I don’t know, I’m a terrible futurist. Every prediction I make is horribly wrong. So I’m not going to go down that road. But we need to be fully aware of what’s going on, learn how to use these tools and learn how we can make our jobs better, and stay ahead of the curve so that we’re not replaced by them.
Jacob Hollabaugh: Yeah, absolutely. And I love the agriculture analogy is one I’ve heard before and one I’ve repeated to people before because I think it does such a great job, of illuminating just how scary it is when we see the headlines or read the blog posts or whatever that say up upwards of 60, 70, 80, whatever per huge percentage of jobs could be replaceable at some point in the future. And we see that number and think, how in the world can we come back from that? If 80% of our jobs ten years from now are fully replaced? How what what does that even mean? And to be able to actually look back and say, well, that level has happened before and we are ignoring the other half of the equation of we figured out a way and things and that new things cropped up, bad pun and not, I guess, kind of intended there since we are referring to farming, but and so I, I’ve used that a couple times myself and had people realize like, oh yeah, I never we just kind of it’s so big and so scary that we don’t think about the other side of things. But that last bit, what you said, is very true for anyone out there.
Jacob Hollabaugh: Just try. Even if you don’t have a direct use case for what these types of tools could be doing for you in your job, learn to use them. I think you know, Gary V is one who’s really big on the idea of just like, no matter what, just like use one every day for something, even if it just goes on Midjourney and make a make a piece of art for no reason other than just get more accustomed. Be someone who is used to writing prompts for AI using the different tools because eventually, they are going to be in. Drained and almost everything we do. And you want to be on the front lines of that to some degree of knowing how to so that the day comes when it’s not just a potential, but kind of a requirement or almost a necessity to be able to do your job the way you know, the efficient, most efficient way you’re ready and able and able to able to do that. The final thing I want to ask you about then here to close out is the implementation of these can be really difficult. That’s kind of where the difficulty lies. You know, for companies especially leaders of companies thinking like, I do want I want to take that advice. You just said, Jacob, I want everyone to either be using it for a benefit to them and their job, but if not to get used to it. So when the benefits do come, they’ll be ready. But I don’t know where to start. I don’t know exactly how other than just saying I want you all to use it. I now go like that doesn’t really that doesn’t really do anything. And so implementation at a company-wide level is very difficult. The implementation at an individual level can be very difficult, difficult. And you wrote a great blog back, I think it was in November, late November. So a month or two ago now about kind of laying the groundwork for AI within your business. So for that CEO or the company, who knows they want to integrate these tools but don’t maybe know where to start? Could you just share a thought or two on some of those important first steps to go to not be the one that just has an all-company meeting and says, use AI go, and actually has something to tell them to start the process?
Glenn Hopper: Here I think 2024 is going to be a big year of FOMO for non-enterprise companies. so all in the way, like I said earlier, maybe 50 million down, but down to your solopreneurs. you’re hearing about all these enterprise businesses that are rolling out. We’re using AI for this and that and that. And you’re you have this sense of, well, I’m never going to catch up with that. And then you don’t have the teams that they do, to run a department like this. But really, what 2024 is going to be about is reskilling and upskilling your team. Like you just said, get familiar with the technology. And the analogy I always use on that is if you don’t understand, I’m not saying you have to be a computer developer or a data scientist, but if you don’t understand at a fundamental level how these models are working, you might as well ask a question and shake up one of those magic eight balls and get the response. Because how do you know if it’s like, what is the answer based on? So understanding the foundational technology. But really the models are only as good as the data that they’re able to use and that they’re trained on. So where I’m telling everyone to focus right now is on their own company’s data maturity. What data do you have? Is it you are their information silos, or is everything being done in spreadsheets? And there’s no single consolidated data lake or data warehouse, where this data can be accessed. What are you doing with the data today? Do you know what the source of truth is for the information that you’re using? So.
Glenn Hopper: And and I think it’s okay, because if you’re not going to develop something internally very extensively to develop these tools internally and it just doesn’t make sense yet. From a cost-to-benefit perspective because the technology’s not quite there, but it’s moving so quickly that I think now is the perfect time. We see what’s on the horizon and it’s coming toward us. So instead of just standing here flat-footed, we can start preparing, start learning the tools that are out there. like your example. Just do something in it every day. Test it. See? See what it gets right, what it gets wrong, where it’s benefits and negatives. Compare the different models go to the, the anthropic one, the Google one, the small models that are out there lama and that and try them out. And, I think familiarizing ourselves with the technology and understanding just the basics of how it works, that’s really where we need to focus this year. but for a company, the most important thing is if you are lagging in your data maturity, then if you’re not catching up now, other companies who have moved ahead of you on that, once there are readily available SaaS type tools that companies can use if you don’t have your data ready, then that’s a whole other time. So now is the time. Get your data house in order.
Jacob Hollabaugh: Yeah. Love it. Well, that’s a great place to end on, Glenn. This has been a real pleasure for those listening who may want to follow you or learn more about Robo CFO, or try out some of the GPTs you’ve created, and keep up with everything you have going on. Where would be the best place for them to go to find you?
Glenn Hopper: I’m fairly active on LinkedIn. the other social media platforms, not so much. Of course, robocfo.ai you referenced my blog there. I need to get I need to be blogging more often there, but I do put a lot of content out on LinkedIn. And certainly, to try out the GPT, you could go there and see them.
Jacob Hollabaugh: Wonderful. We will link to those and more in the show notes below. Glenn, thank you so much for your time and knowledge today. I’ve greatly enjoyed it and hope to speak again sometime soon.
Glenn Hopper: All right. Thank you, Jacob.
Jacob Hollabaugh: If you enjoyed this episode and want to hear more, head on over to soarpay.com/podcast to subscribe on your podcast listening platform of choice. That’s soarpay.com/podcast.