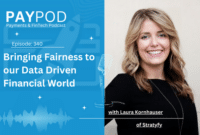
Bringing Fairness to our Data Driven Financial World with Laura Kornhauser of Stratyfy
Episode Overview
Episode Topic:
Welcome to an insightful episode of PayPod. We get into the vital topic of AI Transparency in Financial Inclusion with Laura Kornhauser, CEO and co-founder at Stratyfy, exploring how advancements in artificial intelligence are revolutionizing the financial sector by promoting fairness and accessibility. We uncover the mechanisms and strategies that ensure AI systems in finance are transparent, accountable, and devoid of biases. This discussion is essential for understanding the intersection between technology and equitable financial services, highlighting the role of AI transparency in creating an inclusive financial ecosystem.
Lessons You’ll Learn:
Through the lens of Interpretable AI for Finance, this episode offers a treasure trove of lessons and insights into the importance of AI transparency in financial inclusion, learning how transparent AI practices contribute to fairer financial services. This episode features lessons on identifying and mitigating biases within AI systems, the impact of transparent AI on financial inclusion, and the steps companies like Stratyfy are taking to ensure their AI models promote equity and accessibility. Laura Kornhauser shares her expert knowledge on fostering an environment where financial technologies serve everyone equally.
About Our Guest:
Laura Kornhauser, the co-founder and CEO of Stratyfy, a pioneering company at the forefront of AI Transparency in Financial Inclusion. With a rich background in engineering and a deep understanding of the financial industry, Laura has led Stratyfy to develop innovative solutions that tackle bias and promote fairness in financial decision-making. Under her leadership, Stratyfy has become a beacon for responsible AI usage in finance, ensuring that technology serves as a tool for enhancing inclusivity and transparency. Laura’s work with Stratyfy exemplifies her commitment to leveraging AI to forge a more equitable financial landscape for all.
Topics Covered:
This episode covers a broad spectrum of topics central to understanding AI Transparency in Financial Inclusion, including the challenges and opportunities of implementing AI in financial services, strategies for achieving transparent AI, and the importance of inclusivity in financial decision-making processes. We delve into how Stratyfy, under Laura Kornhauser’s direction, is pioneering efforts to ensure AI systems in finance are both fair and effective. Discussions also extend to the broader implications of AI transparency for regulatory compliance, customer trust, and the future of financial technology. Join us as we explore these crucial themes, shedding light on the path towards a more inclusive and transparent financial ecosystem.
Our Guest: Laura Kornhauser- Leading the Charge in AI Transparency for Financial Inclusion
Laura Kornhauser stands at the forefront of AI Transparency in Financial Inclusion, serving as the co-founder and CEO of Stratyfy. Her journey into the world of finance and technology began with an engineering background, which laid a solid foundation for her pioneering work in the financial sector. After graduating, Laura ventured into banking, where she quickly found her niche. Her tenure at JP Morgan Chase, spanning over a decade in various lending and risk roles, provided her with a unique vantage point on the intricacies of financial services and the critical role of fairness and transparency within them. This experience was instrumental in shaping her perspective on the potential of technology to make financial services more inclusive and equitable.
In the midst of her successful career, Laura identified a pressing need for change within the industry—specifically, the challenge of bias in financial decision-making processes exacerbated by opaque AI systems. This realization prompted her mid-career pivot: resigning from her stable position to pursue an MBA, where she further honed her entrepreneurial spirit and vision for a more equitable financial landscape. It was during this period of exploration and learning that Laura met her future co-founder, Dmitri, and together, they embarked on the journey to establish Stratyfy. The company was born out of a shared mission to leverage AI for good, ensuring that financial institutions could make better, unbiased decisions through transparent and interpretable AI technologies.
Under Laura’s leadership, Stratyfy has emerged as a beacon of innovation in AI Transparency in Financial Inclusion. The company’s unique approach, focusing on interpretability and fairness in AI models, has positioned it as a critical player in the movement towards more ethical use of technology in finance. Laura’s vision extends beyond the immediate impact of her work, aiming to set new standards for how financial services can and should operate in an increasingly digital world. Through her efforts, she champions the cause of financial inclusion, advocating for systems that not only recognize but actively correct biases, thereby opening doors for previously underserved communities. Laura’s work is a testament to the power of technology as a force for good, driving change towards a more inclusive financial future.
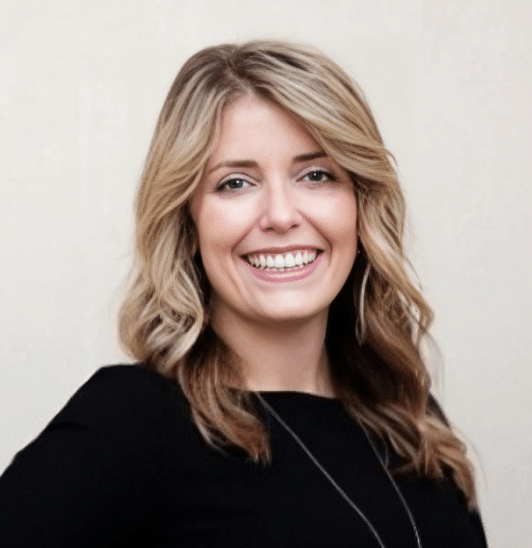
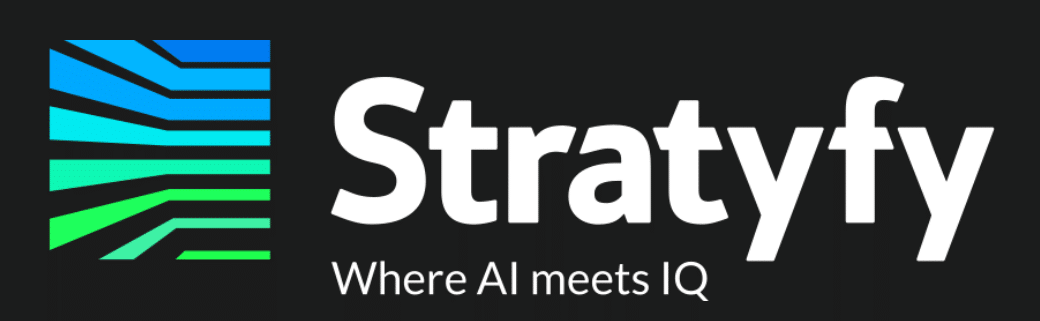
Episode Transcript
Laura Kornhauser: Humans, as we know, have all sorts of both conscious and unconscious biases that lead to different decisions. So, for example, if a certain community or a certain segment of individuals was always denied or was more heavily denied access to fairly priced credit instruments, that then means that they’re forced to go to more predatory alternatives, which then can be ultimately a self-fulfilling prophecy and lead to further worsening of credit. Further, derogatorily, in a credit account, etc.. We’re focused on untangling that, if you will, helping provide visibility to our customers of how and where that bias is permeating into their models and decisioning strategies, and then giving them the control and the tooling to make changes.
Jacob Hollabaugh: Welcome to PayPod, the payments industry podcast. Each week, we’ll bring you in-depth conversations with leaders who are shaping the payments and fintech world, from payment processing to risk management, and from new technology to entirely new payment types. If you want to know what’s happening in the world of fintech and payments, you’re in the right place. Hello, everyone. Welcome to PayPod. I’m your host, Jacob Hollabaugh. And today on the show, we’re talking data analytics, credit risk, fraud, and compliance, all topics we’ve touched on many times before. But today is going to be a little different as we’re going to be diving into how to make these things not just more accurate or efficient, but fair. In other words, how to remove bias from these fields. It’s a topic I’m very interested to get into the how and the why we should be doing this, and I’m very pleased to have an amazing guest to talk about all of this with. I’m joined today by Laura Kornhauser, co-founder and CEO of Stratyfy, the company on a mission to accelerate financial inclusion by providing greater transparency and less bias to critical financial decisions. Laura, welcome to the show. Thank you so much for joining me today.
Laura Kornhauser: Thank you so much for having me, Jacob. I’m excited for our conversation.
Jacob Hollabaugh: Same here. The matter can be fintech, a big wide world, but I always love when we get to talk about a topic that we’ve covered a lot, but a kind of new level to it, or a different look, a different lens to it, which is what we’re doing here today. But before we dive into Stratyfy into all of these things, can we get a quick little kind of overview of who you are, and what your career was like up to the point of 2017? If I have my dates correct, then what kind of ultimately led what you were seeing in 2017 or prior to that that led to the idea for and the founding of Stratyfy?
Laura Kornhauser: Absolutely. My journey before starting Stratyfy started as an engineer. I was an engineer and an undergrad, didn’t necessarily know exactly what I wanted to do after school, but knew I had a lot of interest in financial services, so decided to go into banking and I ended up finding a home in the banking industry. I spent 12 years at JP Morgan Chase in both lending and risk roles, and had a wonderful, as I often jokingly call it, career 1.0 at that fantastic organization. In my time there, quite a number of things, of course, led to my eventual decision to resign in my mid-30s, go back to business school full-time, and pursuits of my entrepreneurial hopes and dreams, which so many thought I was crazy for doing at that time. But what was palatable in my experience, is there was I was responsible for a suite of product offerings. We called our quantitative investment solutions. They were algorithmic trading strategies that were selling to both corporate and institutional customers. We put a ton of effort, time, and money into this new product suite. We’re getting ready to launch it. All of a sudden Dodd-Frank came along. Then these new product offerings that we were getting ready to launch were in the scope of Dodd-Frank regulation. And we hit a brick wall, just an absolute brick wall.
Laura Kornhauser: We needed technology in order to be able to comply with that new regulation that had just come out, which these products were in scope for, and we didn’t have it. We went to our tech team. We said, hey, we need this. As I often joke, they said, we can get to it in Q5 because technology teams and financial institutions, even the biggest ones out there are tremendously, I would say, overworked. We weren’t able to launch this product offering. I ended up working nights and weekends with a colleague of mine to scotch tape something together. It wasn’t pretty, but it got the job done. It meant that we met our product launch date, and we’re still able to comply with the standards of JP Morgan, which are very high from the compliance standpoint with this new regulation. So that was a huge aha for me, that there’s this opportunity at the intersection of financial technology for highly regulated use cases for new solutions. And that’s what started my journey to founding Stratyfy. I come from a family of entrepreneurs, so I’d always had these hopes and dreams of starting a company. Then I was very fortunate that through connections with friends at business school, I met my amazing co-founder Dmitri, and we launched Stratyfy in 2017.
Jacob Hollabaugh: Then tell me a little kind of high level about Stratyfy. We’ll get into the weeds as we go here. But first just a high level. So we all have our bearings. Who is Stratyfy? What’s the service offering and who are you typically working with?
Laura Kornhauser: Absolutely. So Stratyfy is a technology company that provides solutions to financial institutions to help them make better risk-based decisions. And we do that by leveraging an inherently interpretable form of AI or more specifically, machine learning, which I know we’ll get into. So importantly, when we say risk-based decisions, we’re talking about decisions like who to lend to, at what price, and what to investigate for fraud. These are the types of decisions we’re talking about. When we say better, we’re talking about more accurate, more efficient, and fair. That’s a key piece as well, is the fairness side of this and how we can ensure that while we’re leveraging very advanced, very powerful technology, we’re making sure that biases of the past are not embedded into the decisions of the future, and that we have the level of visibility, transparency, control in those systems such that we can correct for those if you will, errors of the past.
Jacob Hollabaugh: Can you give us some kind of concrete examples in the lending world or otherwise, of some of the ways bias has shown up in the past that Stratyfy is working to correct?
Laura Kornhauser: First, it’s important to find what we’re talking about as far as biases. When we’re looking at biases, we’re looking at a variety of biases. But it often comes down to biases against certain protected classes or protected groups. Another bias, though, that we help our customers correct for, is also just bias that is inherent in the fact that the data that you’re using to train a machine learning model is historical. You’re using, if you will, old information, old data, and old results to inform decisions about the future. And that has inherent biases associated with market conditions and other things happening that impacted those results. So that’s another big bias. I often just refer to that as in general, the historical bias associated with data. If we go to the biases across different protected classes, in particular lending decisions for a long time, and many still today are made based on a number of more traditional indicators of creditworthiness that have been proven to be disproportionately distributed across protected classes. So credit score is one that you hear about all the time, and how folks from Bipoc communities have significantly lower average credit scores than folks from white communities, for example. That then leads to lenders who have an overreliance on things like a credit score, which many lenders do, embedding those biases inherent in that credit score or other indicators into their models and their decisions.
Laura Kornhauser: The other thing that embeds these biases into the data that is used to train a machine learning system is the fact that a lot of the decisions that were made by humans and humans, as we know, have all sorts of both conscious and unconscious biases that lead to different decisions. So, for example, if a certain community or a certain segment of individuals was always denied or was more heavily denied access to fairly priced credit instruments, that then means that they’re forced to go to more predatory alternatives, which then can be ultimately a self-fulfilling prophecy and lead to further worsening of credit. Further, derogatorily, in a credit account, etc.. We’re focused on untangling that, if you will, helping provide visibility to our customers of how and where that bias is permeating into their models and decisioning strategies, and then giving them the control and the tooling to make changes. That’s the key. You have to first be aware of the bias, and our technology helps do this, makes you aware of the biases, allows you to track them over time, and allows you to understand the root causes of those biases, but then you have to be able to make those changes. This is the place where we see interpretability being so important in the machine learning space because it gives the user the ability to go inside the box and make changes.
Jacob Hollabaugh: Is that where then you have the unbiased solution that makes this an actual reportable KPI? So some sort of actual number that you could put to it, which is fantastic to see. Without giving away the secret sauce that all the tactical aspects behind it. Can you tell me a bit about how that KPI works and what types of data it is then pulling from, or what types of things, if I’m someone who comes along and uses this, it’s going to be looking at what the score actually looks at and what it’s telling me?
Laura Kornhauser: Absolutely. Our unbiased product is focused on helping lenders automate their fair lending testing, do it in a more rigorous and proactive way, and then have the information that they need to take action when a risk emerges. So we separate out the steps, if you will, or phases of that product into three phases. The first is “Uncover”. So if I have a biased risk, we enable that through automated testing. I can get more into exactly how that works. But automated testing and allowing lenders to run that type of test more robustly and more frequently, then, should a risk emerge, we move to step two, which we call to understand is all about how do I decompose that bias risk that has emerged? How do I know what is driving it? How do I know what factors within my model or decisioning strategies are causing this bias risk that was uncovered? And then three importantly, how do I now take action? That’s what we call undo. So I found a risk I know what’s driving it. Now I want to take action to mitigate that risk going forward. In undo we offer a lender fully customized to their strategies on how exactly they can make changes to their model or decisioning strategy in order to mitigate that bias while sacrificing as little performance as possible. In some cases, you don’t actually even need to sacrifice performance, right? Going back to what you’re talking about earlier about accuracy, Jacob, I think there’s this belief that accuracy and fairness or accuracy even and transparency need to be these two sides of the scale. They don’t have to be.
Jacob Hollabaugh: Can you walk us through a little of how removing that bias actually does improve that accuracy, and improve your ability to make better decisions, and if there are any numbers behind like the industry average versus what your products are able to do once they get through those steps to the other side from an accuracy standpoint?
Laura Kornhauser: Better does mean more fair, but better also means more profitable. Yes, you can have both of these things and you can have them both at the same time, which is even better. The way we’re able to enable that with our technology is by having a richer way that we look at the data that is being used to ultimately calculate the riskiness of a potential borrower and put a more precise number on that riskiness than other methods are able to do. That’s how we drive that better accuracy piece, and we do that while also balancing our world. We think of bias or fairness as a KPI of any model or a decisioning strategy, just like I’m looking at accuracy by typically a variety of different metrics. I should also be looking at fairness metrics when I’m evaluating model A versus model B, or decision strategy A versus decision strategy B, so we illuminate that for customers in our products, and then we’re able to show them that, hey, this fairer model often has accuracy right there with the model that has no, if you will, knowledge of fairness. And then that accuracy if you will show off. Oftentimes, it’s a little drop in accuracy ends up being hypothetical. I’ll explain what I mean by that. When you provide access to folks that have been left out, folks that have been used to unfortunately getting, if you will, the door slammed in their face, one end up having a valuable and loyal customer that you’ve now attracted, and they end up performing a lot better than that historical data or that ultimately biased data.
Laura Kornhauser: The ways we were talking about before would have thought they would have performed. So we find a lot of those individuals that, based on older methods of evaluating riskiness, would have been viewed as high risk based on our methods, and more nuanced and accurate methods of evaluating risk, now show that they’re not as high risk as we thought. They end up performing even better orders of magnitude differences. Jacob, they’re big now. The numbers depend on kind of the starting point of a lender that we’re working with, of course. But just to give you one example of a US-based lender that we worked with that was using more old-school methods for evaluating risk and traditional credit bureau attributes. In order to do that evaluation, we came in, used our system, and were able to drive a 140% increase in loan approvals, while also slightly reducing the expected default rate. It was only by about ten basis points. So not a huge reduction of risk, but a massive improvement in approvals, which translates into a very meaningful bottom-line impact for the financial institution less compliance risk better relationships and engagements with their community, a more loyal customer base that they can cross, sell, and upsell into other products and services that they have at their financial institution. So it can be a windfall for these FIs.
Jacob Hollabaugh: What is the response from those banks or lending companies and all the different customers that you work with? Because we do know about the world right now is much positive talk is there is around issues such as bias. Do you feel like the companies you work with are coming to you putting the same importance? Are they always as interested in the fairness piece as they are just interested in? Are you more or less accurate? What’s the reception to the fairness portion of what you do from your consumer base?
Laura Kornhauser: We find that the reaction and the reception, it varies. When we think about our mission is to drive greater financial inclusion, while also helping FIs better manage and mitigate risk. We see that as two sides of the same coin, we can’t meaningfully move forward with financial inclusion if we’re not showing FIs why it’s good business to do and again, showing that bottom-line impact. We need to do both in order to convince the masses right or get mass adoption. A lot of the lenders we work with are highly mission-aligned with us. They care a tremendous amount about fairness in their decisions. They see what we were just talking about, the long-term value of that, even beyond the individual loan for growing their loyalty with their customer base and growing their reception within their community and their perception within the community. We do have some lenders that we work with that are focused, though, on that bottom line impact or I should say impact to their bottom line, and are want to make sure that they don’t run afoul of regulation. They want to make sure that they’re doing the right thing and complying with regulations, and that’s very important to them. But the key driver for them is the business value is the bottom line impact. That’s why we’re so focused on both sides of this coin and making sure that we’re delivering both from our customers, because that’s the way we know we can drive the biggest impact as a company, as a technology provider, to these financial institutions.
Jacob Hollabaugh: Let’s go back to the idea of AI and machine learning and what you are doing with that. And all the way back really, to the founding of the company first. It feels like you were maybe a little early, if not straight up, just early on. Applying AI to this industry and to products that nowadays are much more obvious use cases. If anyone was starting a company in this world and wasn’t doing it based off of AI, we would probably all, what are you doing? How are you expecting to compete? But when you were starting the company, I’m guessing it was a little bit more new. Talk to me about those early days. Did you ever face any confusion from potential customers, or any pushback from folks that maybe weren’t ready early on for machines doing a bigger part of this versus the human side of everything?
Laura Kornhauser: This is where I think our competitive differentiation in the machine learning space is so powerful and so impactful. When we were starting the company, one of the things that we were seeing out there in the market was there was a lot of talk about machine learning and the value that it could deliver to financial institutions, but that value was not being unlocked. There was a lot of talk, maybe not as much talk as today about AI, but there was a lot of talk about it, and especially from the technology community, there was a lot of talk about machine learning and how it could help revamp processes like credit underwriting to drive more accurate predictions of risks and better decisions. But what we found, and we knew this from our own experiences. All of our management team has a deep background in financial services and intimate knowledge of how banks work and the challenges banks face. So we saw that while there was a lot of hype in the technology space when you went to the adoption, it fell off. We would see a lot of machine learning technology was being piloted or being tested, but it ran into a lot of roadblocks or brick walls when it went to be implemented or productionalize. A lot of times those brick walls were because of model risk management, and other internal governance and compliance concerns, all relating to the black box problem of a lot of other machine learning systems. This is a known problem. So much so now that probably the second most popular buzzwordy term behind AI is transparency, which everybody claims to have. We are very focused on the distinctions between different levels of transparency and what exactly is meant if you will, the grouping of very different levels of transparency into this one-like headline of, oh, we’re not a black box, we’re transparent.
Laura Kornhauser: What we mean by transparency is not just the ability to fully understand what happens in the box. So full understanding of everything that happens in the box. We’re not adding post-hoc explainers. You see everything that’s going on. Then the second level is you have the ability to make changes. And I think this is so crucially important. It’s crucially important in the area of bias mitigation in particular, but also in other areas where data is not representative or a perfect sample of what you believe will happen in the future, which I would argue is everywhere. If you don’t have that level of transparency that gives you that control to go in and make changes to compensate, your data is not fully reflective of how you want to make decisions going forward, either for embedded biases, historical biases, or for other reasons. We feel that our approach, which falls into the category of interpretable machine learning, is the right approach to get greater adoption in financial services. That’s what I think helped us break through a lot of that early clutter in our early days, Jacob, where there was a lot of hype, but not as much action, especially in production, and not as much value as a lot of AI or machine learning projects were falling on the floor after a pilot that shows great results, and then we try to deploy it and we can’t or those results don’t hold true once we go into deployment, or all the other concerns that have plagued AI and machine learning in financial services for a while.
Jacob Hollabaugh: The final question then, to get you out of here on Laura is what are you most excited about for the year ahead? Are there any big plans we should be expecting from Stratyfy, or any big trends that we haven’t talked about? Are you trying to stay in front of, or is there anything in the remaining year ahead that is the most exciting for you?
Laura Kornhauser: Two things I would love to highlight here, Jacob. So one is we are the technology partner for an initiative called Underwriting for Racial Justice, where we’re working with 20 lenders, all of whom are committed to making proactive changes to their underwriting strategies to drive greater access for Bipoc communities. It’s an unbelievable group of forward-thinking, mission-driven lenders, and we are so honored to be the technology partner that is helping them, not just figure out what changes they should make and what those changes will look like and get those changes implemented, but also collect and share insights across the group such that learnings from one FI can help better the other 19. Rising Tide raises all of the boats. I think it is a landmark initiative, one that I have not seen in the industry before as far as the commitment level and the coordination across different financial institutions. So we will start this year. We will start getting results from that program, which we have a very high expectation for, and I couldn’t be more excited for us to share more broadly, to help convince or motivate. More lenders to join us in that activity. So that’s one I’m super excited about. The other thing I’m excited about for us ahead is as there is more AI and machine learning technology getting adopted by financial institutions, there are more concerns about biases that are inherent in these systems and understanding exactly how these systems work.
Laura Kornhauser: We have a unique technology competitive advantage, a lot of experience in helping MFIs manage through those challenges and help them illuminate and take a proactive response or proactive action to address these types of concerns, address these types of risks, and keep on innovating but innovating in a responsible way. That’s the key. There’s a ton of value that AI technology can deliver to financial services and other industries, but it’s important that’s done in a responsible way. And we believe that sustainable and accountable technology is so important in that, and you can’t be accountable if you’re not transparent. I’m very excited for the massive step function that I think we will see this year on, in general, the adoption of AI technologies and then the need to prove to yourselves, to external parties, to your customers that there aren’t inherent biases baked into those, and to truly understand how those systems are working so you can have confidence in them. Right. It’s very hard to have confidence in something that you fundamentally don’t understand. We can’t be reliant on only data scientists to understand these things. We need to make sure that we can communicate across stakeholders, across folks with different levels of familiarity with AI, with technology in general, in order to have the level of impact that we all know this technology can have on financial institutions, on their customers, on financial inclusion, on all of it.
Jacob Hollabaugh: It’s amazing work you’ve already done and wonderful work in the future for you. For anyone who wants to keep up with some of those projects you mentioned, or just learn more about Stratyfy, or maybe get in touch or follow you, where’s the best place for them to go?
Laura Kornhauser: LinkedIn is a great place to follow our company. Also, feel free to reach out to me directly to connect. I’d love to meet folks listening. You can also check out our website www.stratyfy.com. That’s stratyfy. Check out stratyfy.com to check out what we’re doing.
Jacob Hollabaugh: Awesome. We will link to those and more in the show notes below. Laura, thank you so much for your time and knowledge today. I’ve greatly enjoyed it and hope to speak again sometime soon.
Laura Kornhauser: There’s a lot of fun! Jacob, thank you so much for the opportunity.
Jacob Hollabaugh: If you enjoyed this episode and want to hear more, head on over to soarpay.com/Podcast to subscribe to your podcast listening platform of choice. That’s soarpay.com/podcast.